For those responsible for Business Architecture and Innovation, one of the most critical points is to establish a scalability strategy and the KPIs/OKR's measure and ensure its success.
In addition to applying RPA (Robotic Process Automation) technologies from start to finish, complementary technologies must be integrated that accompany the efficiency and effectiveness of the final result.
On the other hand, punctual and non-continuous tactical solutions can be prioritized, but always taking into account a long-term global roadmap. An exclusively tactical approach runs the risk of producing a lack of credibility and trust within the organization that views those responsible as apagafuegos without continuity or strategy (see profile WOLF).
The main challenges that can be found when creating a global process efficiency and effectiveness plan are:
- Lack of guidance and strategy to help organizations integrate process automation (RPA) systems with other tools. This hinders the end-to-end vision of the processes, and therefore strategic commercial values are lost.
- Initiatives to add artificial intelligence to business processes often occur in silos without an overarching strategy embedded in their developments. This leads to future volume scalability, technological and organizational design issues.
Therefore, to accelerate business transformation and the new architecture it is recommended to:
- Plan a long-term strategic roadmap aligning business objectives, identifying processes to optimize and choosing complementary technologies to the process.
- Develop an integration strategy that enables end-to-end automation and helps design the RPA, business process management (BPM), and other necessary tools.
- Augment business and customer relationship processes through the progressive integration of artificial intelligence to tap into long-term business value niches.
By 2022, 65% of organizations that have integrated robotic process automation will introduce artificial intelligence, including machine learning and natural language processing algorithms.
Introduction
Business architects (EAs) and technology innovators in general are often pressured from business to focus on the fast, tactical needs of process automation.
This approach can provide quick relief as a form of non-invasive integration. However, the processes are not always simple, routine, repetitive and stable. They can be long-lived and often involve intelligent, automated decision-making and optimization. The real challenge is to have a vision of scale beyond the few initial fruits of routine processes. This cannot be solved with a single tool or isolated strategies.
La hyperautomation refers to the effective combination of complementary tool sets that can bridge functional and process silos to automate and augment business processes.
This hyperautomation is based on a set of tools to simplify, measure and manage processes throughout the company. Among these tools can be found, beyond RPA, BPM engines, workflow systems, decision management suites, process mining, low code application platforms (LCAP), among others.
The first question that arises is where to start to scale correctly. This document proposes an approach in three phases.
- Analysis: Define the journey towards automation.
- Co-create a strategy to combine digital technologies and operations.
- Augment business processes with AI.
For each of these steps, it is necessary to take into account the axes of customers, technology platforms, ecosystems and the resulting products. Counting in all the axes with the motors of Intelligence and Artificial Intelligence.
Analysis: Defining the journey to automation
Defining the roadmap is the first step. It is important to establish the desired business result and the processes that need to be optimized, before automating and assembling the rest of the complementary tools.
Expected Business Results
Sales
Costs
Risks
Process Optimization
Evaluate / Dimension
Industrialize and scale
Continuous improvement
Integration with Internal Tools
Tools
iPass
Artificial Intelligence
Expected Business Results
It is key to define the digital objective when automating any process.
Architects and business managers must collaborate to establish a future vision of the results differentiating between Transformation and Optimization: cost reduction, customer satisfaction, agility, sales, etc. These objectives can be grouped into three groups:
- Income. what are the key takeaways for revenue? For example, you may choose to focus on improving processes, automating tasks, increasing customer engagement, or introducing new services.
- Costs.: Does it drive cost optimization? You can improve efficiency by automating tasks, but also by redesigning processes, reducing the cost of errors and speeding them up.
- Risks. What are the compliance, regulatory or legal risks due to inefficient processes? By redesigning and automating processes, the risk of non-compliance must be minimized.
Identify use cases that you want to optimize to improve the effectiveness and efficiency of a process. Aims to transform business processes
experimenting with new ways of generating value. This can be done by redesigning and optimizing processes. In your roadmap, clearly distinguish your use cases and outcomes associated with your revenue, cost, and risk goals.
Process optimization
Very often, automating a bad process can make it worse. It is essential to structure business processes to achieve high performance. This is applicable when automation requires organizations to take a fresh look at key process characteristics related to data structure, component delimitation, and exceptions.
Identify use cases to optimize processes by focusing on:
- Adjust the process intelligence quotient. Determine how "intelligent" the process should be from the point of view of its consumers.
- Industrialize and scale the core processes that drive the products and services delivered through a digital business platform (APIs, service ecosystems, third parties, etc.)
- continuous improvement by process with structured, standardized results and intelligence for decision making.
Integration with Internal Tools
The tools that are part of the automation process are multiple and varied. It is necessary to identify those that are closely aligned with the planned roadmap.
Therefore it is important to evaluate different technologies and create a progressive investment plan to effectively deliver tactical and strategic business value.
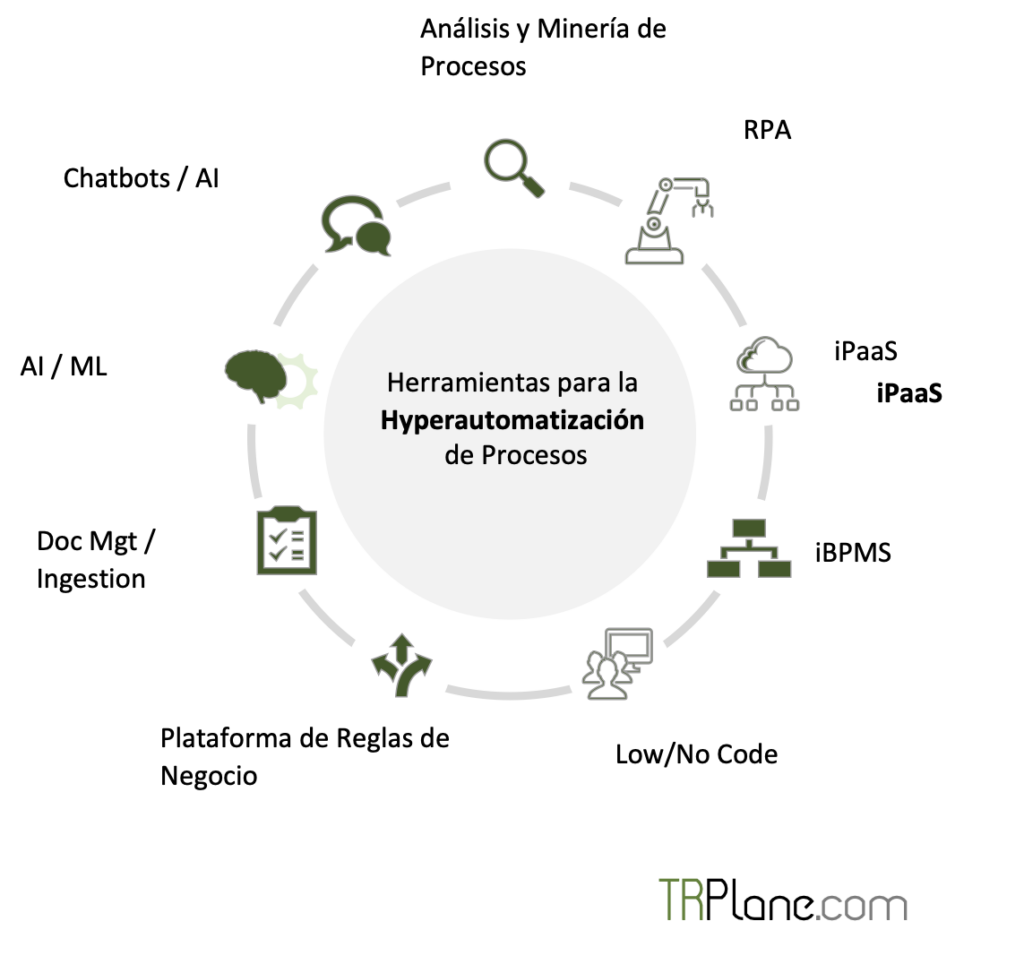
A set of tools aligned with business-driven process automation provides various options to address the different steps of process automation (discover, analyze, design, automate, measure, monitor and re-evaluate).
Evaluating use cases and long-term business goals helps identify the optimal combinations of these tools.
However, it is not possible to claim to cover all of them, even if they are available, since the analysis phase can take a long time and lead to paralysis. Some of which are not included without APIs and Enterprise Service Buses (ESBs).
BPM / iBPMS Platforms
Intelligent BPM suites (iBPMS) are a mature set of tools on the market for orchestrating processes and automating tasks within those processes. iBPMS consolidate integration services, decision management, process orchestration, ad hoc processes, and advanced analytics on a single platform
Managing a BPM/iBPMS tool involves functionally focusing on:
- Manage long-term processes that cross the entire organization that include people, hardware services, software and rules or functional limits.
- Apply iBPMS as a master orchestrator for processing and managing task lists.
- Activation of a robot or RPA script to automate a task with a process. APIs can be used to integrate RPA scripts with the BPM Master Orchestrator.
- Monitoring of metrics and creation of analysis tables to identify opportunities to improve the process.
- Provide direct integration services / APIs to other platforms, applications and devices in the organization or outside it.
RPA
RPA is a non-invasive integration technology used to automate routine, repetitive and predictable tasks through orchestrated user interface interactions that emulate human actions.
Using an RPA functionally encompasses:
- Fast deliveries and business benefits by automating routine and repetitive tasks.
- Creation of interfaces with APIs with other applications. This non-invasive means interacts with applications, generally developed in the past, in which the construction of the traditional integration would be complex, slow and expensive.
- Obtaining, consolidating and validating data from different sources in a large-scale migration project.
- Quickly experiment with prototypes. Applying RPA allows for quick concept tests before launching a new service or process.
Low Code / No Code Application Platforms (LCAP)
LCAP environments often have a powerful graphical front end and can therefore be used to model rapid automation of a process. Most LCAP providers offer business process orchestration and workflow services to quickly automate tasks and streamline them into simpler processes.
When applying the LCAP, the following aspects must be taken into account:
- It is typically optimal for processes with small scope or that are frequently changed and limited to a single function or team within the organization. The ability to quickly go from idea to running application is critical to LCAPs. This functionality puts LCAPs in a very strong position to automate business processes that need a high degree of flexibility.
- Create or modernize the user experience (UX) of a process that requires a web or mobile user interface. Many LCAPs are also cross-platform development platforms.
- Take advantage of the rich set of connectors to automate tasks related to applications that have existing APIs in the organization. However, the set of supported protocols is smaller than that of an integration platform or BPM suite. Connectors in LCAP are primarily focused on HTTP REST API-based connectivity or connectors for regular enterprises and SaaS (such as ERP systems).
Process mining and discovery/analysis
Process mining is aimed at discovering, monitoring and improving real processes by extracting knowledge from the event logs available in application systems. Process mining includes automated process discovery, compliance checking, and other advanced analytics.
These tools apply to:
- Identify process efficiencies at a granular level.
- Discover, monitor and configure tasks that can be automated by bots / scripts.
- Extract knowledge from events available at the workstation or captured from screens.
- Create process documentation and automatic generation of simulation models.
- Solution or extension of the models.
- Make process recommendations based on historical data.
These tools can be integrated with BPM and RPA platforms to optimize routine automation opportunities by discovering, monitoring, and analyzing business processes, tasks, and interdependencies.
Decision Management Suites (DMS) / Business Rules Management Systems (BRMS)
DMSs are used to complement conventional application development when applications involve decisions involving complicated or frequently changing logic. Modern DMS products have evolved beyond business rules management systems by providing better support for decision modeling and analysis. These can be used in conjunction with BPM tools.
A DMS is relevant to applications that include decisions involving any of the following scenarios:
- Various types of input data, which can be collected from various sources.
- multiple calculations
- Dozens, hundreds of thousands of trade policies, implemented as rules
- Sophisticated algorithms involving a mix of analytical rules and models
- Multiple stakeholders, sometimes with different goals
When integrating a DMS platform, the following must be taken into account:
- Creation of own decision model: for example, mixed conceptual and logical models.
- Improve authoring or decision maintenance tools, for example, through metaphors that make it easier for the company to create or modify rules and analyzes without the help of complex technological developments.
- Supported predictive analytics scoring services, such as the ability to import or connect with PMML, PFA, R, Python, or other models from data science platforms (or similar advanced analytics tools).
Approach and challenges
Those responsible for business architecture have the challenge of transitioning from a
loosely coupled RPA ecosystem with some technologies, to a seamlessly connected set of tools. This concept is being given the name of Digital Ops. As of 2020, many providers provide a single platform with an integrated offering. For example:
- Microsoft has launched the Power Automate solution as RPA with its Power Apps low-code and workflow applications.
- SAP launched its integrated BPMRPA offering from its enterprise cloud, integrated with S/4HANA ERP.
- pegasystems already provides an integrated BPMRPA solution.
- Appian It has partnered with UiPath, AutomationAnywhere (very famous and one of the first and most versatile RPA on the market) and Blue Prisma.
- Oracle has partnered with several RPA providers.
The transition from a model where an RPA is available with a series of various complementary tools (Complemented RPA, CoRPA) is clearly different and evolves towards a model of architecture and availability of DigitalOps tools.
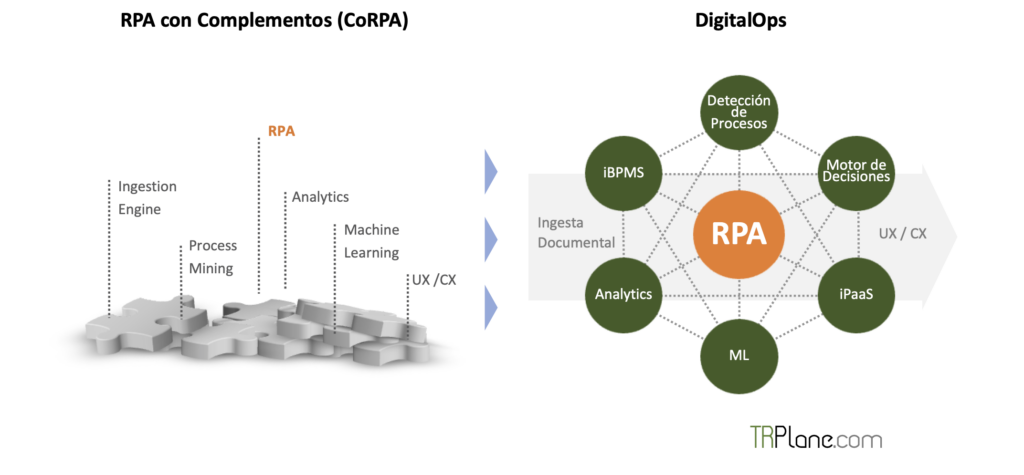
Augment and optimize business processes using AI
To accelerate hyperautomation, an integrated intelligence system effectively combines the tools of Digital Ops with:
- artificial intelligence (AI)
- Machine learning (ML)
- Natural Language Processing (NLP)
- Optical Character Recognition (OCR)
- conversational chatbots
An integrated model of AI, ML and NLP could have the following functional architecture:
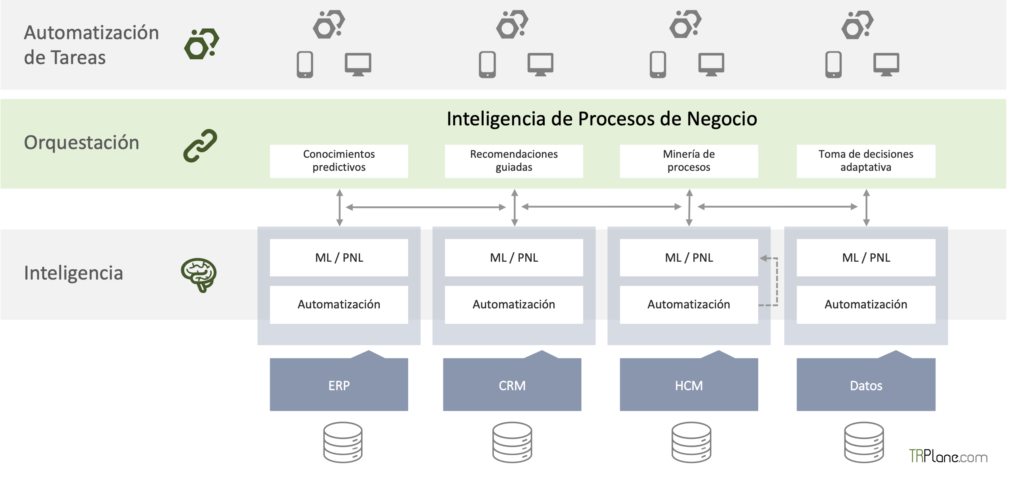
In an integrated ecosystem of DigitalOps tools:
- RPA allows the automation of tasks.
- BPM / LCAP / DMS enables dynamic orchestration.
- The AI layer adds intelligence to the process.
Existing transactional ERP and customer-centric platforms incorporate their own local process-centric tools.
AI can be applied at every application integration layer to facilitate predictive insights and enable guided recommendations, process mining, and adaptive decision making.
A business process that affects many applications and systems can effectively use AI, ML, and NLP models to accomplish the same thing.
AI and ML are mostly used in automation scenarios to:
- Perform continuous learning with the data collected in automation processes to dynamically update models, which improves the quality of automation, and make adjustments accordingly.
- Reuse training models (i.e., models trained for other clients for use cases). use similar and then reused in a new client model). This minimizes the time and data required to build and deploy a model.
- Protect model privacy by ensuring that any client information used to train the model is extracted from reused training models.
- Automate the training and construction of ML models without requiring the manual process of training and selecting ML algorithms.
Common use cases for AI, ML, and NLP within business process automation include many industry examples, such as:
- Claims handling in insurance (eg, extraction, classification of claims data)
- Anti-money laundering efforts in banking (e.g. reconciliation, transaction data monitoring)
- Contract management
- Legal processes
- Clinical trials and pharmacovigilance in healthcare
- Automated query/support selection and resolution
To achieve business value, solutions must be implemented that deliver specific and measurable business results for each use case. Discovering and developing candidate AI and ML use cases as a team allows you to identify measurable business outcomes from each of these use cases.
Knowing how the AI functions within the automated process will work with other components is key. If decisions need to be justified within a process, then the use of AI and ML may not be a good approach, or some form of human oversight may need to be integrated.
Example 1
The aim is to automate the process for the prevention of money laundering. implementing a fraud detection algorithm, all integrated with the current systems of the organization and non-routine tasks, intelligence for decision making and human judgment.
This would involve the following elements and steps:
- An intelligent business process management suite and/or distribution management system tool manages the workflow/process orchestration according to various decisions.
- Activate an RPA bot / script to perform data collection and other validation routines on customer, market, supplier, etc. data.
- The fraud detection algorithm, built on top of an ML model, which runs on the consolidated data to identify patterns. This process could introduce human intervention, requiring a formal approval or electronic signature to be provided in order to proceed.
- Later another RPA to carry out follow-up actions, such as sending emails and updating transactional systems (such as the ERP solution, CRM and other internal applications).
Example 2
Other routine tasks are usually found in the relationship with customers through contact centers.
- Use an NLP text analytics feature to perform customer sentiment analysis based on transcripts of customer complaints.
- Identify the plan to prepare the agent and systems to drive value-added conversations.
- An attended RPA bot performs follow-up actions for the agent, such as preparing a structured email with valuable data for the customer.
Recommendations
The use of AI, ML and NLP has several relevant aspects to take into account to obtain the best result in your application.
- Identify the most relevant motivations for the use of each area of AI, including ML, NLP, OCR and chatbots.
- Assess the necessary resources, skills, time, costs, and complexity involved in building AI models to justify each business case.
- Ensure the availability of good quality historical data in ML models.
- Analyze all factors, trigger points, subsystem limits, interface APIs, exception handling, and edge cases where human intervention is required.
- Plan for minimum viable, fast and iterative deliveries in business operations.
- Exploit AI accelerators from leading cloud service providers that can integrate with proprietary LCAP, DMS, BPM, RPA, and iPaaS platforms. Common AI/ML libraries often available on these platforms include AWS Machine Learning, Google Cloud (TensorFlow), IBM Watson Studio, Microsoft Azure Machine Learning Studio, StanfordNLP, Natural Language Toolkit, and spaCy.
- Include AutoML features to enable RPA processes to optimize ML and NLP accelerators. AutoML engines use input and output from completed manual tasks to select algorithms, train models, and push models to automation without interruption.
Acronyms
AI | Artificial Intelligence |
BPM | Business Process Management |
EA | Business Architects |
DMS | Decision Management Suites |
iBPMS | Intelligent Business Process Management Suites |
iPaas | Platform as a Service integration |
LCAP | Low-Code Application Platforms / No-Code Application Platforms |
ML | Machine Learning |
NLP | Natural Language Processing |
OCR | Optical Character Recognition |
RPA | Robotic Process Automation |
SaaS | Software as a Service |